Thanks to artificial intelligence (AI), new technologies have been implemented, able to provide improvements and additional information with respect to the simple, even if essential, counting of people. With the new sensors for people counting it is indeed possible to obtain:
the staff exclusion;
the identification and counting of buying groups;
the gender recognition (male or female).
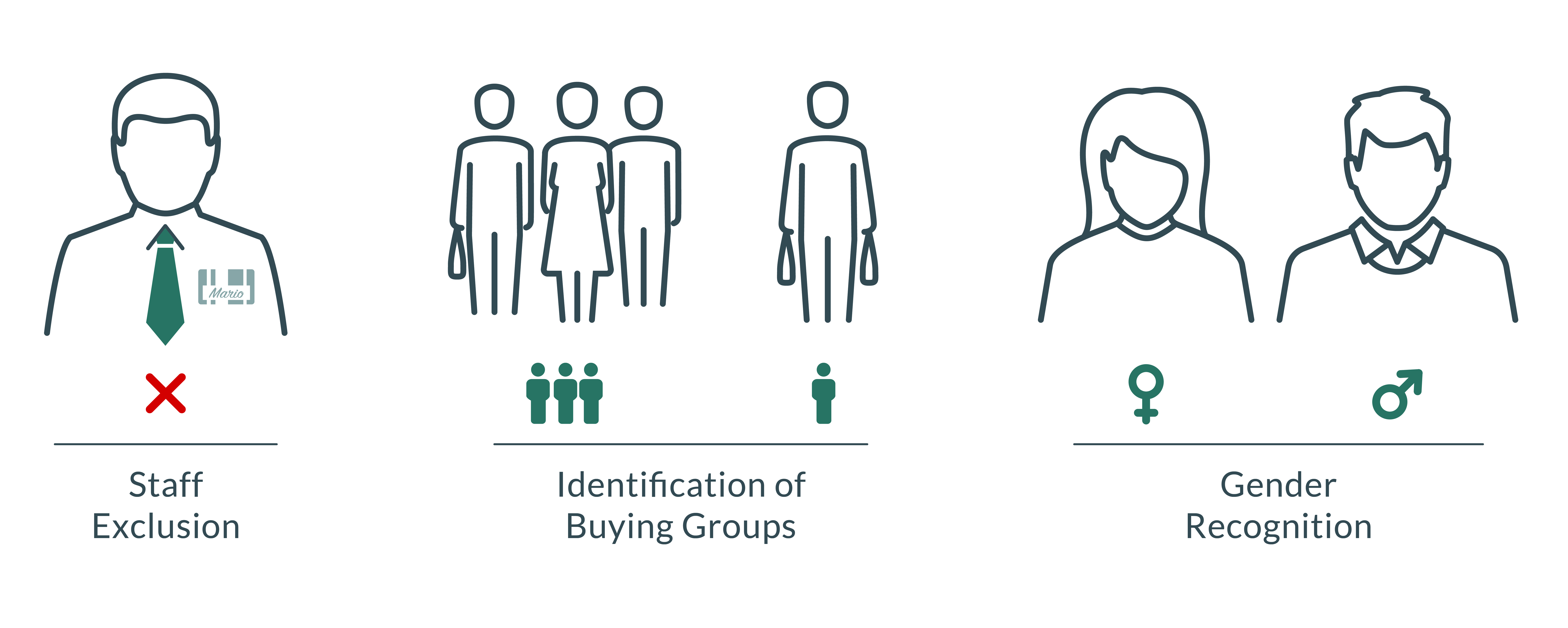
To monitor the performance of a commercial reality open to the public, the footfalls remains the fundamental metric.
However, according to Microlog, it is important to keep up-to-date on the current possibilities, in order to consciously choose the most suitable people counting system according to the information you desire to obtain, or to evaluate an upgrade of an already existing system. To make true data-driven decisions, data quality is the best investment!
Exclusion of staff and identification of buying groups. The new sensors with AI on board are able to avoid the counting of the people who wear a small tag (more or less as big as a business card), customizable in color and in the name of the employee. Thus, it will be enough to ask the salespeople to put on this mark and it’s done: only prospects customers will be counted.
Thanks to the recognition of the directions, the relative distances and speeds of people, the new people counting systems are able to identify those who, with a high probability, belong to the same purchasing group, such as to a family. Typically, the average number of members of a group during the weekend is greater than that during weekdays, as the greater availability of free time makes it more likely to go shopping in groups rather than alone. This is how the conversion rate (ratio between the number of receipts and people leaving) of the weekend tends to be lower than what it should be if compared to that of weekdays. The explanation of this phenomenon could lie in less sales performances, but also be due exclusively to the natural tendency for a group to merge purchases of several people into a single ticket, and/or to be accompanied by someone when going shopping. Calculating the conversion rate with buying groups rather than with individuals helps to have a more reliable sales performance indicator.
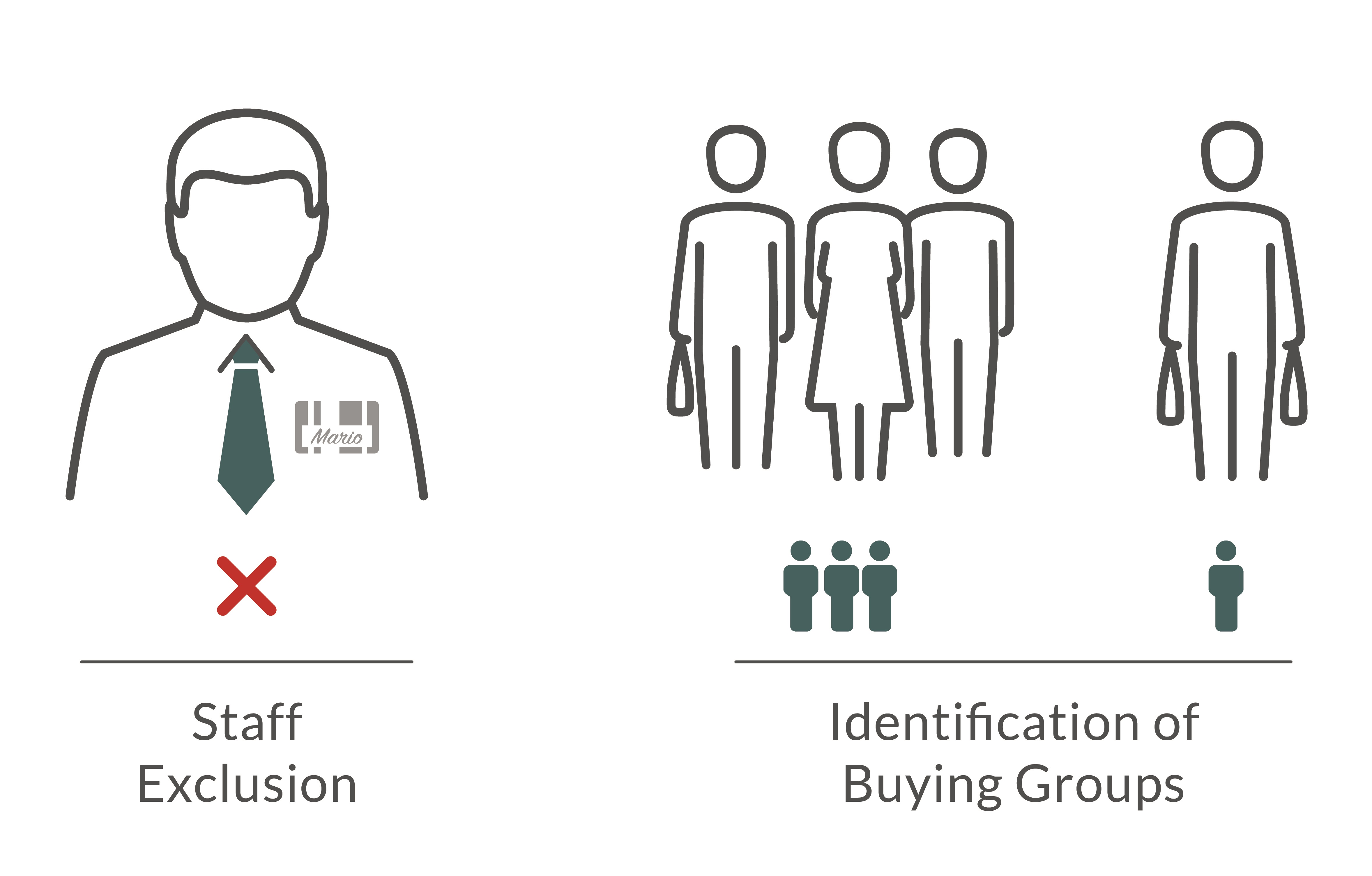
With the exclusion of staff and the recognition of buying groups, it is therefore possible to obtain the footfalls without the passages of the salesmen, as well as a conversion rate more comparable between weekdays and weekends, i.e. a conversion rate more informative about sales performance.
To all this, it is possible to associate a filter that limits the count to people above a certain (customizable) height. The aim is to exclude younger children, who have no purchasing power and therefore do not represent potential customers.
Gender: how? The people counting system. If the exclusion of staff and the recognition of groups are tools for improving the quality of the data, the average number of members of the purchasing groups begins to provide some additional information about customer behavior.
But it is with the recognition of the gender that the new people counters offer real profiling data, useful for knowing customers.
Thanks to the AI, the system is able to recognize, from appearance and movements, the gender of a person who is entering or leaving. The purpose of the sensor is to classify the person as male or female, and to return the percentage of male and female on the total. In the rare cases of indecision, in which a sufficient degree of confidence on the gender of a person is not reached, the classifier avoids attributing a gender, rather than having a too high risk of error.
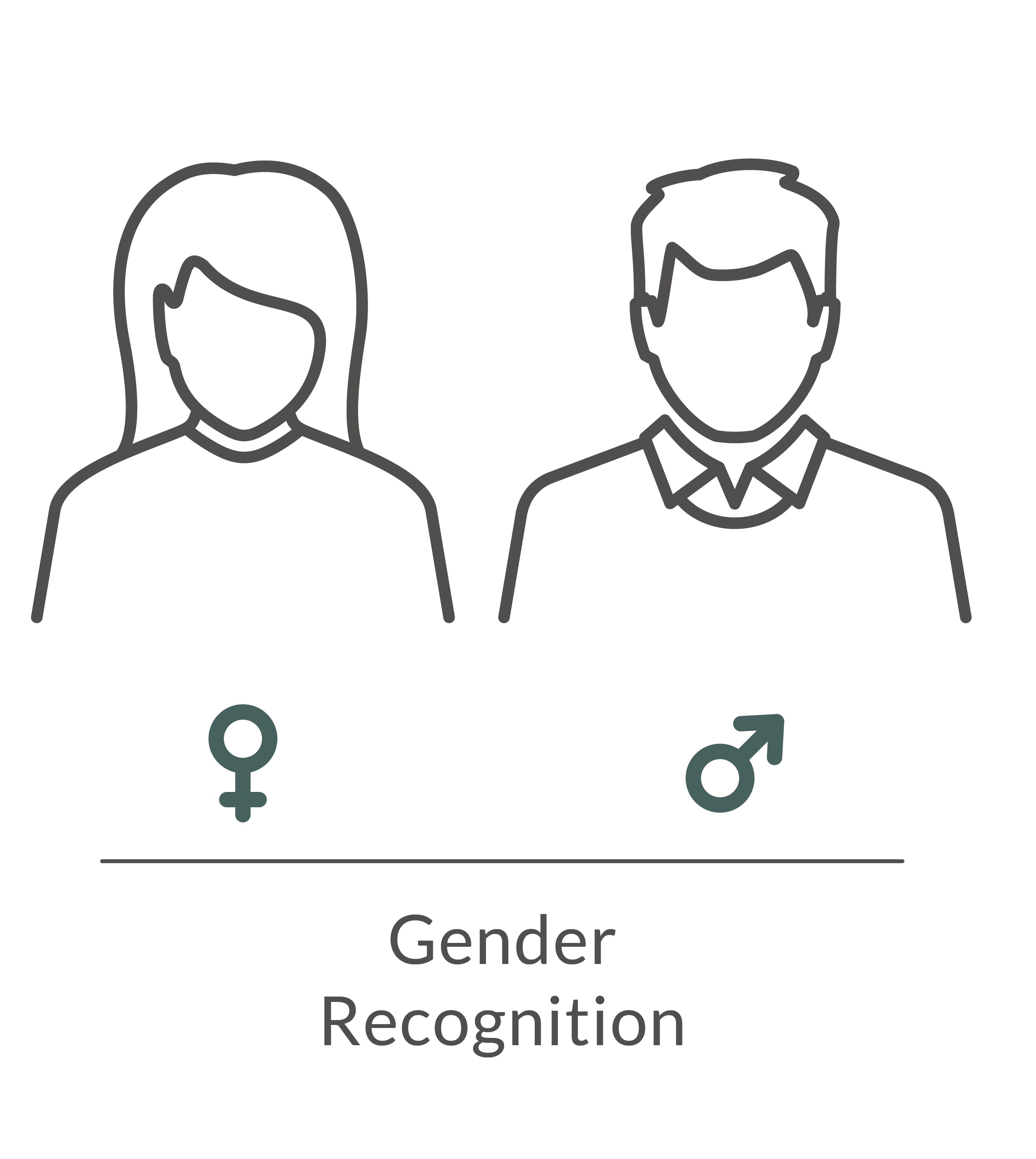
Gender: why? Gender marketing. Why would the data on male and female be useful? Men and women are different: researches show that male behaviors, needs, preferences and decision-making processes are different from those of women. The effects of these differences also occur in the buying habits. As a matter of fact, there is a branch of marketing called gender marketing.
It is important to highlight that the gender represents not a person’s biological sex, but his/her socio-cultural gender. The gender marketing applies notions of psychology and neuroscience to adapt the advertising and non-advertising communication to the buying behaviors of a gender.
It could be argued that, if the purchasing habits between gender were particularly different years ago, now they are less marked: it is no longer just women who buy household items, they are no longer just men who do bricolage.
Yet, men and women tend to be different in their preferences, needs and way of thinking. Rely on these differences or on common features could be a winning choice.
The following table shows some of the ideas offered by gender marketing, which moves from the definition of male and female “typical behaviors”.
Man | Woman | |
---|---|---|
Conception of shopping | Shopping as a mission: the man goes shopping to buy what he needs. | Shopping as a journey: the woman goes shopping to find out what she needs. |
Decision making process | Linear process: the man addresses each phase only once. He is always focused on his starting need and, step by step, he takes the final decision. His goal is to find a good solution, that is a product suited to his needs. | Spiral process: the decision phases can be repeated each one several times. The goal of the woman is to find a perfect solution with respect to a detailed list of criteria. This list can be modified at will, even during the purchase process itself, which becomes longer and more complex than that of man. If a woman finds a good solution, this may not be enough to finalize the purchase: she seeks perfection, even if it implies to visit more stores and having to spend more time. |
Environment | It must be functional, organized to find everything quickly, with few distractions. The purpose is to stay there only as long as necessary. | It must be welcoming, designed to be a place to stay for long and pleasantly; it must be studied in detail, full of ideas. |
Type of purchase | Goal oriented. The man knows what he needs: he arrives, buys and goes away. Impulsive: “… nice the product on the promotional expositor! I want it!” (Without evaluating other possibilities) |
Thoughtful: “I am not convinced at all, perhaps in the shop X they have something better …” |
Communication | Concise and clear advertising messages, with assertive and direct sentences, in animated and competitive contexts. | Advertising messages that convey in sentimental and emotional ways, in contexts of real family life. Better to avoid peremptory messages such as “come and visit us right today”. |
Obviously, there are women who have buying behaviors or preferences more similar to those described above for men, and vice versa. This is not to be thought of as “wrong”: each person is unique as she/he is. However, researches show that applying the indications of gender marketing works: on a statistical basis and without the expectation of being able to adapt to every single human being.
Knowing the percentages of men and women it is possible to know, by comparison with the local census data, if you are attracting a particular segment of the population.
It is therefore possible to work in order to induce those you have already attracted to purchase or to address the less represented segments of the population.
There are many possible strategies based on gender. Some of these are the following:
making the most from the different purchasing habits, for example taking advantages of the (typically male) impulsive purchase through the products near the cash desk;
changing the environment, which must be functional for men, cared for women;
proposing targeted advertising and promotions (using special tools, such as totems);
monitoring how the male/female composition varies according to events (by consulting the data on hourly basis), in order to identify those that attract men or women.
Exclusively for Shopping Centers and Outlets it is also possible to perform the following actions:
changing the store mix, in favour of those male-oriented or female-oriented;
place differently the stores, in order to create associations or male/female oriented zones;
monitoring the variations in male/female composition in response to modifications in the stores mix, not only in the overall Mall/Outlet but also in specific zones or levels.
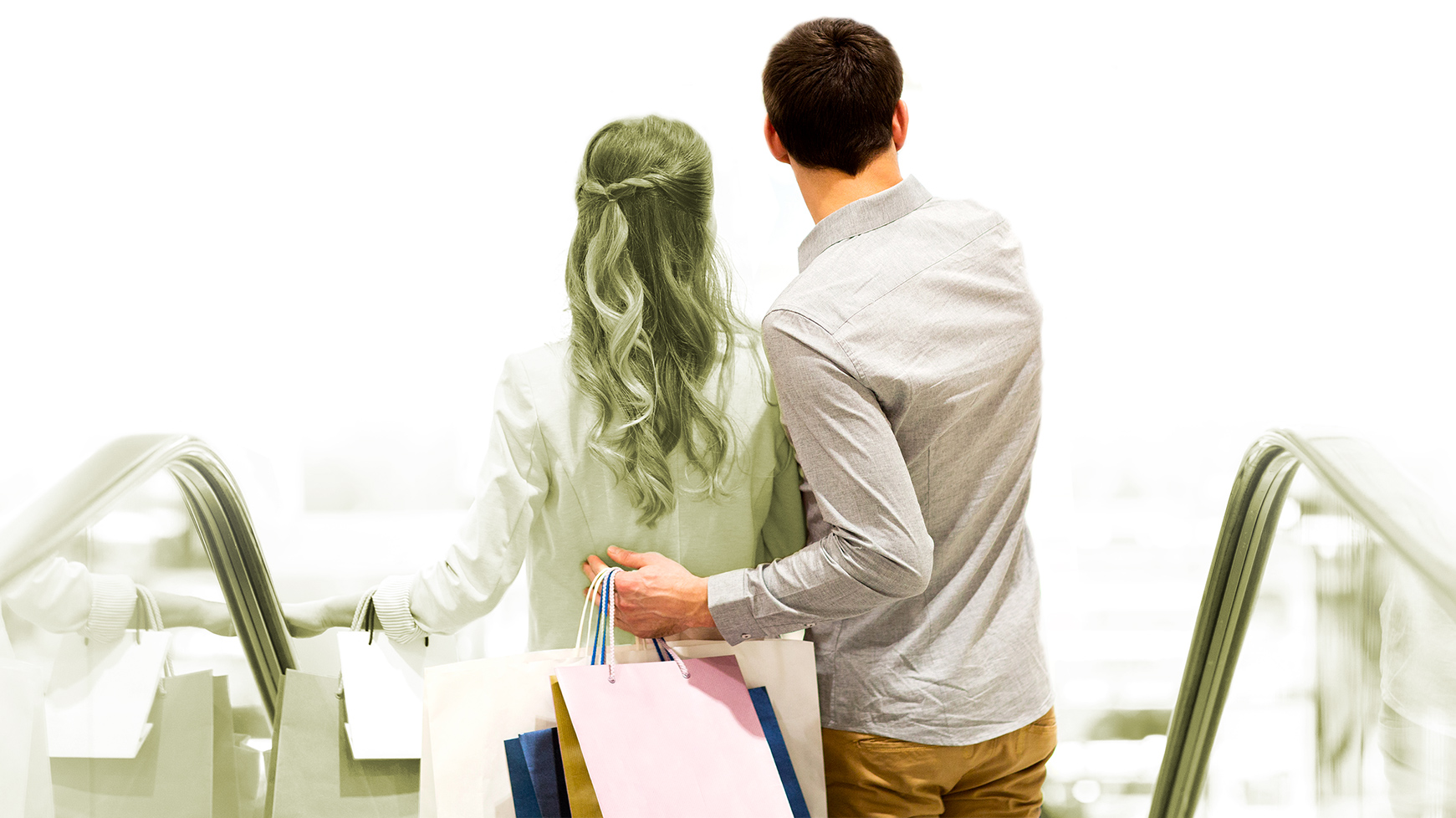
In terms of profiling customers, further scenarios are to combine the data about gender with those about age (collected with surveys or with dedicated services, such as a captive portal) and to estimate average shopping time and average value by gender.
Gender: really? Microlog’s tests. But how good are these data? What is the performance of the classifiers? Before proposing these new solutions, Microlog has tested them, with the awareness that it is useless to have data if you are not convinced of their quality: the data-driven strategic decisions are effective only if the data are reliable.
In the following we report the data of a real test, in order to give an idea of the goodness of the classification. The following tables show:
the percentage error the sensor made in counting;
the detection rate (i.e. the percentage of people that the sensor was able to classify as male or female);
the analysis of the classifier, carried out on the classified people.
People in | People out | |
---|---|---|
Error in counting | 1,2% | 0,8% |
% of detection | 95,7% | 97,0% |
Legend of confusion matrix:
Male, classified as male | Male, classified as female |
Female, classified as male | Female, classified as female |
Classifier (people in):
108 | 2 |
1 | 135 |
accuracy (correct classifications over the total of the classifications) |
98,8% |
M correctly classified over the total of the classifications M | 99,1% |
F correctly classified over the total of the classifications F | 98,5% |
M correctly classified over the total of the true M | 98,2% |
F correctly classified over the total of the true F | 99,3% |
% male (reality) | 44,7% |
% female (reality) | 55,3% |
% male (classifier) | 44,3% |
% female (classifier) | 55,7% |
Cohen’s kappa coefficient performance of the classifier |
97,5% |
Classifier (people out):
104 | 1 |
0 | 125 |
accuracy (correct classifications over the total of the classifications) |
99,6% |
M correctly classified over the total of the classifications M | 100% |
F correctly classified over the total of the classifications F | 99,2% |
M correctly classified over the total of the true M | 99,0% |
F correctly classified over the total of the true F | 100% |
% male (reality) | 45,7% |
% female (reality) | 54,3% |
% male (classifier) | 45,2% |
% female (classifier) | 54,8% |
Cohen’s kappa coefficient performance of the classifier |
99,1% |
If you need more information about the new features presented and/or if you are interested in the possibility to implement new people counting systems or to upgrade existing ones … feel free to contact us! ?
Write a comment (Your email will not be published and will remain confidential)